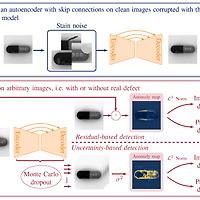
1. Abstract [산업 현장에서 AE를 활용한 Anomaly Detection] Industrial vision에서 Anomaly Detection problems은 결함이 있거나 없는 arbitrary image를 clean image에서 mapping하도록 훈련된 AutoEncoder를 사용하여 해결할 수 있습니다. [skip-connections이 있는 AutoEncoder(AES)를 사용한 이유] 이 접근 방식에서 Anomaly Detection과정은 개념적으로 본다면 reconstruction residual 또는 reconstruction uncertainty에 의존합니다. 공통적으로 sharpness of the reconstruction를 높이기 위해 skip-connections이 ..
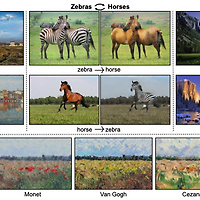
1. Abstract Image-to-image translation is a class of vision and graphics problems where the goal is to learn the mapping between an input image and an output image using a training set of aligned image pairs. However, for many tasks, paired training data will not be available. We present an approach for learning to translate an image from a source domain X to a target domain Y in the absence of ..
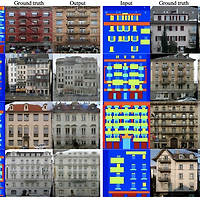
1. Abstract image-to-image translation problems에 대한 일반적인 방법부터 Contitional adversarial networks를 사용한 방법까지 조사했다. Image-to-Image translation problems를 다루는 Networks는 입력 이미지에서 출력 이미지로 가는 mapping을 학습할 뿐만 아니라 loss function도 학습한다. 이를 통해 image-to-image translaotion problems에서 각 상황에 따라 사용되는 loss functions이 달랐지만 paper에서 제안한 방식을 적용하면 동일한 loss functions을 사용하여 적용할 수 있다. paper에서 제안한 방법을 사용하면 label maps에서 사진을 합..
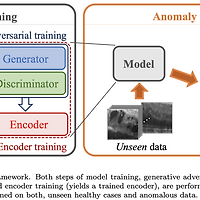
1. Abstract [문제점] 정확한 annotation은 시간이 많이 들기 때문에 전문가(방사선사, 의사 등)가 clinical imaging(임상 영상)을 직접 annotation을 표시한 데이터를 얻는 것은 어렵다. 또한 모든 병변에 대해 annotation이 표시되지 않을 수도 있으며 annotation에 대해서 정확하게 이 병변이 어떤 병변인지 설명 되어있지 않은 경우도 있다. [Supervised Learning의 장단점] 전문가로부터 분류된 training data를 받아 Supervised Learning 방식으로 모델을 학습 시키면 좋은 성능을 얻는 반면, annotation이 표시된 병변으로만 제한이 되는게 단점이다. [Unsupervised Learning으로 접근한 f-AnoGAN..
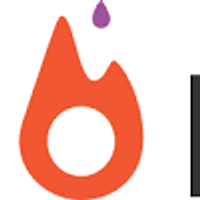
이번엔 Pytorch를 사용하면서 데이터, 학습 과정 등의 시각화에 유용한 TensorBoard를 사용하는 방법을 한번 알아보도록 하겠습니다. 1. Install 우선 TensorBoard는 이름 그대로 Tensorflow를 위해서 개발된 Tools입니다. 그래서 Tensorflow에서만 거의 사용이 되었지만 정말 감사하게도 Pytorch에서도 TensorBoard를 사용할 수 있도록 TensorBoardX라는 라이브러리를 개발을 해주셔서 이제는 Pytorch에서도 Tensorboard를 사용할 수 있게 되었습니다. TensorboardX를 사용하려면 pip을 사용해 TensorboardX를 설치해야 합니다. pip install tensorboardX 2. Sin, Cos Function을 그려보기. ..
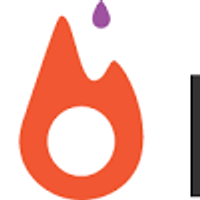
Tensorflow 1.x 버전으로 코드를 작성하다가. Naver AI HackaThon을 계기로 Pytorch로 넘어오게 되었다. 어떻게 보면 비슷하지만 약간은 다른 Pytorch를 그동안 접하면서. 초보자들에게 유용한 팁을 한번 간단하게 정리를 해보고자 한다. (이미 잘 하신 분들은 스킵하셔도 됩니다~~) 글의 구성은 다음과 같이 구성이 되어있습니다. Custom Dataset 을 Class로 만들어서 Data Loader 시키기. Transfer Learning and fine tunning with Pytorch Save and Load pickle file Model feature extractor 1. Custom Dataset Loader Pytorch에서 기본적으로 제공해주는 Fashion..
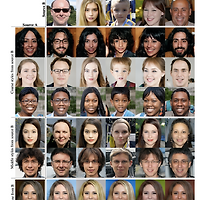
1. Abstract We propose an alternative generator architecture for generative adversarial networks, borrowing from style transfer literature 라고 논문 초반 부분에 나와있다. 해당 논문에의 핵심은, Generator Architecture를 중심적으로 설명하고, 이 Architecture를 기존에 하던 방식(PGGAN)과는 다른 방법으로 구성을 했다라는게 핵심 포인트이다. 또한 Image에 Style을 scale-specific control하게 적용 했다 라고 주장을 하고 있다. 그럼 여기서 Style을 scale-specific control하게 적용한 의미가 무엇인지 한번 짚고 넘어가보자. Q..
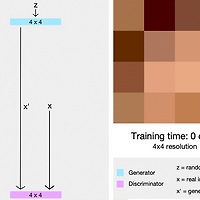
1. Abstract 1-1. Problem PGGAN(Progressive Growing of GANs)은 2017년도에 나온 Nvidia에서 나온 논문이고 발표 당시 큰 이슈를 받았다고 한다. 그 이유는 그 전까지 GAN들은 고해상도(High resolution) 이미지를 만드는것이 매우 힘들었는데 이 논문이 새로운 접근 방법으로 고해상도 이미지 생성하는 것을 해결했다. 일반적인 GAN으로 고해상도 이미지를 만든다고 한다면, 아래와 같은 문제들이 발생을 할 것 이다. - 고해상도 이미지를 만드는 것은 매우 어려운 일이다. 그 이유는, High Resolution 일수록 Discriminator는 Generator가 생성한 Image가 Fake Image인지 아닌지를 구분하기가 쉬워지기 때문이다. - ..