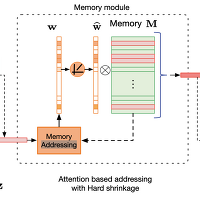
1. Abstract 정상 데이터를 이용하여 Autoencoder(AE)을 학습하면 정상보단 비정상 데이터에 대해 더 높은 재구성 오류(reconstruction error)을 얻게 됩니다. 하지만 AE는 일반화(Generalization)가 잘 이루어진다는 특징이 있어서 비정상 데이터가 입력되어도 정상을 재구성 해야하지만 결함이 있는 부분까지 포함하여 재구성 하는 경우가 발생하게 됩니다. 이런 AE기반 이상 탐지(Anomaly Detection)의 한계점을 개선하기 위한 해결책으로 메모리 모듈(memory module)을 사용하여 AE을 augmented 하는 방법인 MemAE을 이 논문에서는 제안하고 있습니다. 방법은 (1) 입력 x가 주어지면 MemAE는 먼저 Encoder을 통..
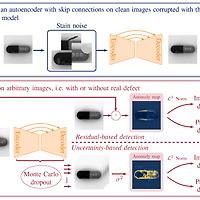
1. Abstract [산업 현장에서 AE를 활용한 Anomaly Detection] Industrial vision에서 Anomaly Detection problems은 결함이 있거나 없는 arbitrary image를 clean image에서 mapping하도록 훈련된 AutoEncoder를 사용하여 해결할 수 있습니다. [skip-connections이 있는 AutoEncoder(AES)를 사용한 이유] 이 접근 방식에서 Anomaly Detection과정은 개념적으로 본다면 reconstruction residual 또는 reconstruction uncertainty에 의존합니다. 공통적으로 sharpness of the reconstruction를 높이기 위해 skip-connections이 ..
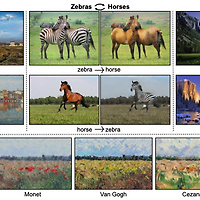
1. Abstract Image-to-image translation is a class of vision and graphics problems where the goal is to learn the mapping between an input image and an output image using a training set of aligned image pairs. However, for many tasks, paired training data will not be available. We present an approach for learning to translate an image from a source domain X to a target domain Y in the absence of ..
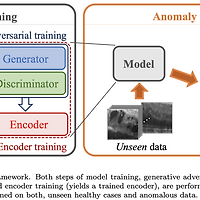
1. Abstract [문제점] 정확한 annotation은 시간이 많이 들기 때문에 전문가(방사선사, 의사 등)가 clinical imaging(임상 영상)을 직접 annotation을 표시한 데이터를 얻는 것은 어렵다. 또한 모든 병변에 대해 annotation이 표시되지 않을 수도 있으며 annotation에 대해서 정확하게 이 병변이 어떤 병변인지 설명 되어있지 않은 경우도 있다. [Supervised Learning의 장단점] 전문가로부터 분류된 training data를 받아 Supervised Learning 방식으로 모델을 학습 시키면 좋은 성능을 얻는 반면, annotation이 표시된 병변으로만 제한이 되는게 단점이다. [Unsupervised Learning으로 접근한 f-AnoGAN..
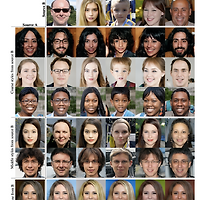
1. Abstract We propose an alternative generator architecture for generative adversarial networks, borrowing from style transfer literature 라고 논문 초반 부분에 나와있다. 해당 논문에의 핵심은, Generator Architecture를 중심적으로 설명하고, 이 Architecture를 기존에 하던 방식(PGGAN)과는 다른 방법으로 구성을 했다라는게 핵심 포인트이다. 또한 Image에 Style을 scale-specific control하게 적용 했다 라고 주장을 하고 있다. 그럼 여기서 Style을 scale-specific control하게 적용한 의미가 무엇인지 한번 짚고 넘어가보자. Q..